⭐ 最新课程+助教招聘
o 机器学习与因果推断专题
2024/11/9-10, 16-17日 (四天)
线上直播, 司继春 || 张宏亮
>> 点击报名 | 助教招聘-10 名
o 选题征集,点击 此处 或者扫码填写问卷留下您的宝贵意见,感谢!
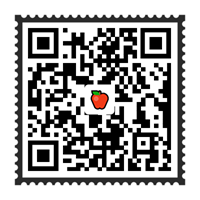
NEW!视频课堂:lianxh-class.cn
⚡ 新版 lianxh 命令:使用说明
. ssc install lianxh, replace
. lianxh 多期DID
⚡ ihelp
:帮助文件 PDF 版
. ssc install ihelp, replace
. ihelp xtreg
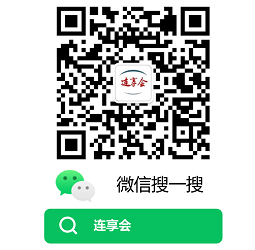
⭕ 录播-因果推断实用计量方法
o 60 天回放;15 位助教全程答疑
o 嘉宾:邱嘉平教授 (加拿大麦克玛斯特大学)
o 课纲:网页版;PDF 版,-报名-