- 时间: 2025 年 5 月 2-4 日
- 方式: 线上直播+30天回放
- 嘉宾: 连玉君;申广军;董展育;林文炼;杨海生 (中山大学)
- 资料: 课程主页 | PDF 课纲 | 文献
- ⛳ 点我报名 | 助教招聘
FAQ:往期课程板书和答疑文档
选题征集,点击 此处 或者扫码填写问卷留下您的宝贵意见,感谢!
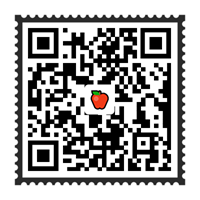
NEW!视频课堂:lianxh-class.cn
⚡ 新版 lianxh 命令:使用说明
. ssc install lianxh, replace
. lianxh 多期DID
⚡ ihelp
:帮助文件 PDF 版
. ssc install ihelp, replace
. ihelp xtreg
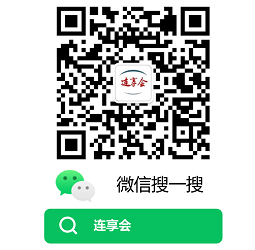