本文基于微软研究院开发的机器学习因果推断的 Python 第三方库 EconML 和应用案例,展示双重机器学习方法的使用。
⚡ 最新课程:
A. 2025 暑期班:连玉君+张宏亮
B. 社会网络分析:杨张博
C. 政策优化和机制分析:杨海生
D. AI+学术研究专题:杨阳
E. ⚡ 系列公开课
- 共八次:Python环境配置; 大语言模型应用; IV 估计; 微观数据清洗; 中介效应 (查看)
- 参与方式: 网络直播 (7.3-24 日),扫码进群即可参与
FAQ:往期课程板书和答疑文档
选题征集,点击 此处 或者扫码填写问卷留下您的宝贵意见,感谢!
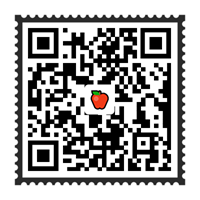
NEW!视频课堂:lianxh-class.cn
⚡ 新版 lianxh 命令:使用说明
. ssc install lianxh, replace
. lianxh 多期DID
⚡ ihelp
:帮助文件 PDF 版
. ssc install ihelp, replace
. ihelp xtreg
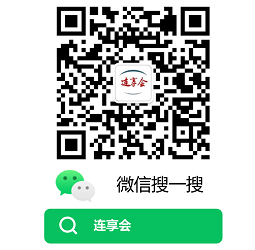