⚡ 最新课程:
A. 2025 暑期班
- 时间: 2025 年 8 月 4-14 日
- 方式: 线上直播+30天回放
- 嘉宾: 连玉君 (初级+高级) || 张宏亮 (论文班)
- 资料: 课程主页 | PDF 课纲 | 文献
- ⛳ 点我报名 | 助教招聘
B. 社会网络分析
⚡ 系列公开课
- 共五次:Python环境配置; 大语言模型应用; IV 估计; 微观数据清洗; 中介效应 (查看)
- 参与方式: 网络直播 (7.3-24 日),扫码进群即可参与
FAQ:往期课程板书和答疑文档
选题征集,点击 此处 或者扫码填写问卷留下您的宝贵意见,感谢!
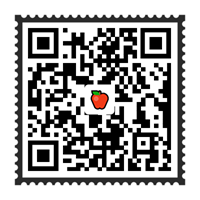
NEW!视频课堂:lianxh-class.cn
⚡ 新版 lianxh 命令:使用说明
. ssc install lianxh, replace
. lianxh 多期DID
⚡ ihelp
:帮助文件 PDF 版
. ssc install ihelp, replace
. ihelp xtreg
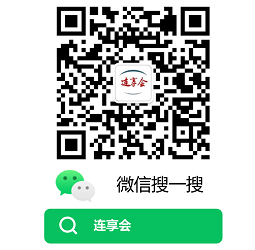